Nowadays, everyone wondering about how they can leverage machine learning in retail. So, you are not the only one. It should be no surprise that artificial intelligence (AI) and machine learning (ML) considerably impact the retail industry, especially for businesses that rely on online sales, where AI technology is already very common.
It is impossible to consider ML and AI separately in today’s technologies. Are you scared of AI jargon? We have already created a detailed AI glossary for the most commonly used artificial intelligence terms and explained the basics of artificial intelligence as well as the risks and benefits of artificial intelligence for organizations and others. So, it’s time to explore the effect of machine learning in retail.
What is machine learning in retail?
In order to process large datasets, identify pertinent metrics, recurrent patterns, anomalies, or cause-and-effect relationships among variables, and thus gain a deeper understanding of the dynamics guiding the industry and the contexts where retailers operate, machine learning is used in the retail industry.
Machine learning algorithms improve their performance when they find new correlations and better structure the business environment they are investigating as more retail data is processed.
The Fortune Business Insights study highlighted how machine learning might be used to create forecasting models and provide insightful data on ever-shifting consumer preferences. With a predicted increase from $5.84 billion in 2021 to $18.33 billion in 2028, machine learning is now retail’s largest subset of the worldwide AI market.
You don’t have to be “big ones”
The entire sales cycle, from storage logistics to post-sale customer care, has been successfully integrated with AI by major players like eBay, Amazon, and Alibaba. To benefit from the immense power of Machine Learning, you do not need to be a large firm or only conduct business online.
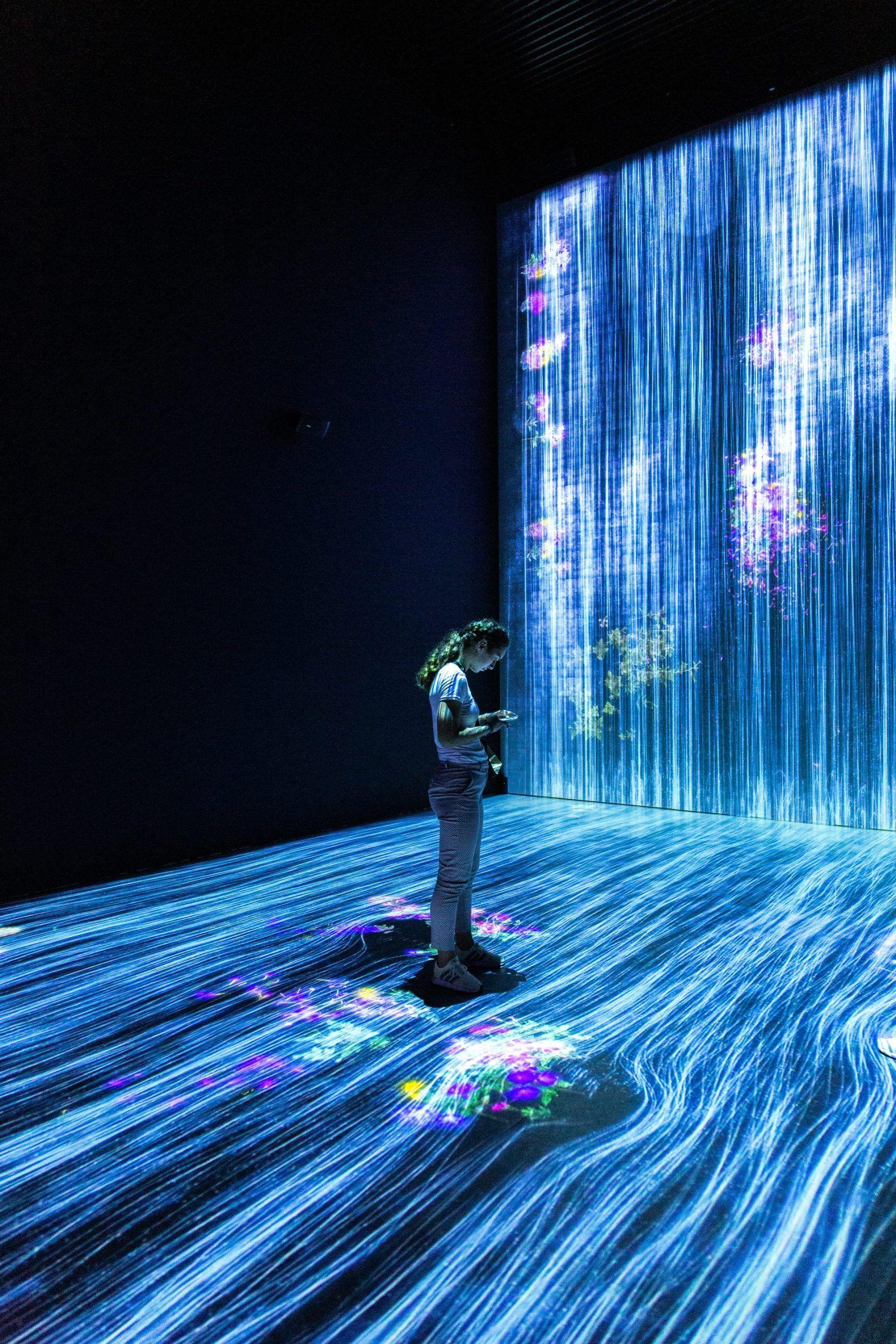
The retail industry offers many opportunities, from clothing to groceries to home goods. Many businesses have requirements that data analysis and tailored machine learning development could meet.
Why machine learning is important or useful in retail?
With the help of little to no human intervention, computer systems may evaluate and learn from data using machine learning.
A machine learning model for retailers may quickly review and convert a large amount of complex data into insights that can be used to:
- Accurate forecasting of upcoming needs.
- Improvement of inventory control.
- Identifying consumer needs through appropriate segmentation.
- Making product offerings more unique.
- Decide on the optimum prices to increase sales.
Check out the effect of artificial intelligence on manufacturing
Are you wondering about the all advantages of machine learning in retail? Keep reading.
Benefits of machine learning in retail
With the help of this technology, you may boost operational effectiveness, cut inventory costs, and modify retail operations to account for current and potential future market trends.
These are the benefits of machine learning in retail:
- Product pricing
- Forecast of the orders
- Personalized 24×7 customer services
- Finding best deals
- Customized shopping experience
- Tracking customer habits
- Staff-less stores
- Wider data access
- Security
Check out how machine learning can drive retail success
However, if you want the advantages of machine learning, you must ensure that your data is accurate and reliable. The cleaned data allows for precise forecasting and the best decision-making while maintaining customer satisfaction.
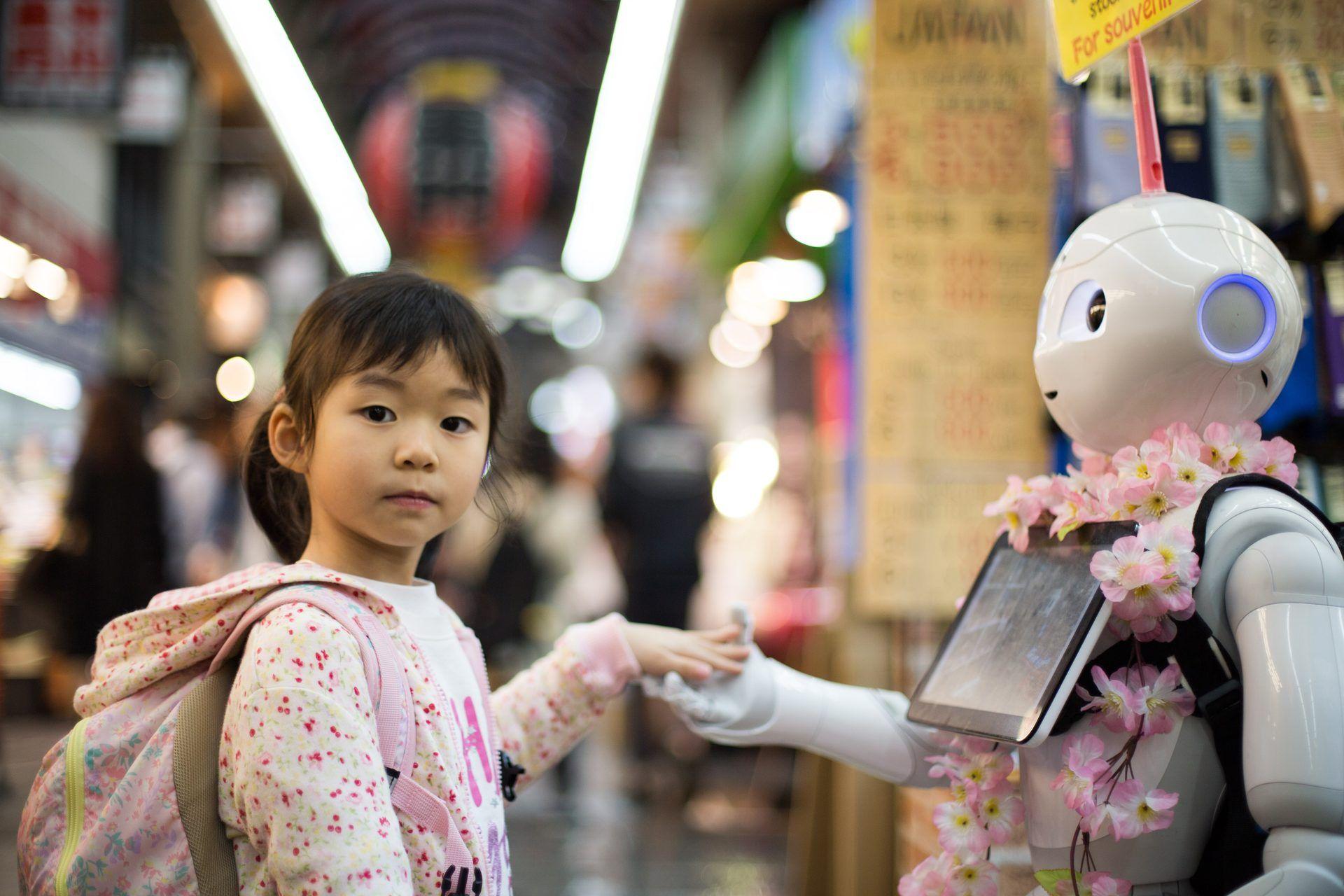
Machine learning in retail use cases
Machine learning is being used by numerous businesses to enhance the customer experience and boost sales. These are the most common machine learning in retail use cases:
- Auto-pricing
- Price optimization
- Demand prediction
- Customer segmentation
- Logistics support (Supply chain management)
- Personalized offers
- Predictive analytics
- Market basket analysis
- Churn rate prediction
- Location optimization
- Classification of customer reviews
- Fraud detection
- Determining customer lifetime value (CLTV)
- Document work automation
- Cross-sell prediction
- Merchandising
Check out some of the retail industry’s machine learning application cases below:
Auto-pricing
Every content published on a website must be priced by a human, which affects costs and publication speed. This is known as the human bottleneck. A person finds it challenging to consider all the variables that affect demand and price.
Machine learning algorithms can automatically and accurately price each product by analyzing data. The model uses image recognition technology to comprehend how the product appears while considering other factors, including brand, fabric, category, description, historical sales data, and previous pricing selections for comparable items.
Price optimization
You can see the market as a whole because machine learning algorithms evaluate a lot of data. AI technologies, for instance, allow merchants to confidently determine whether any of their resellers breach the minimum advertised price by tracking the behavior of their resellers.
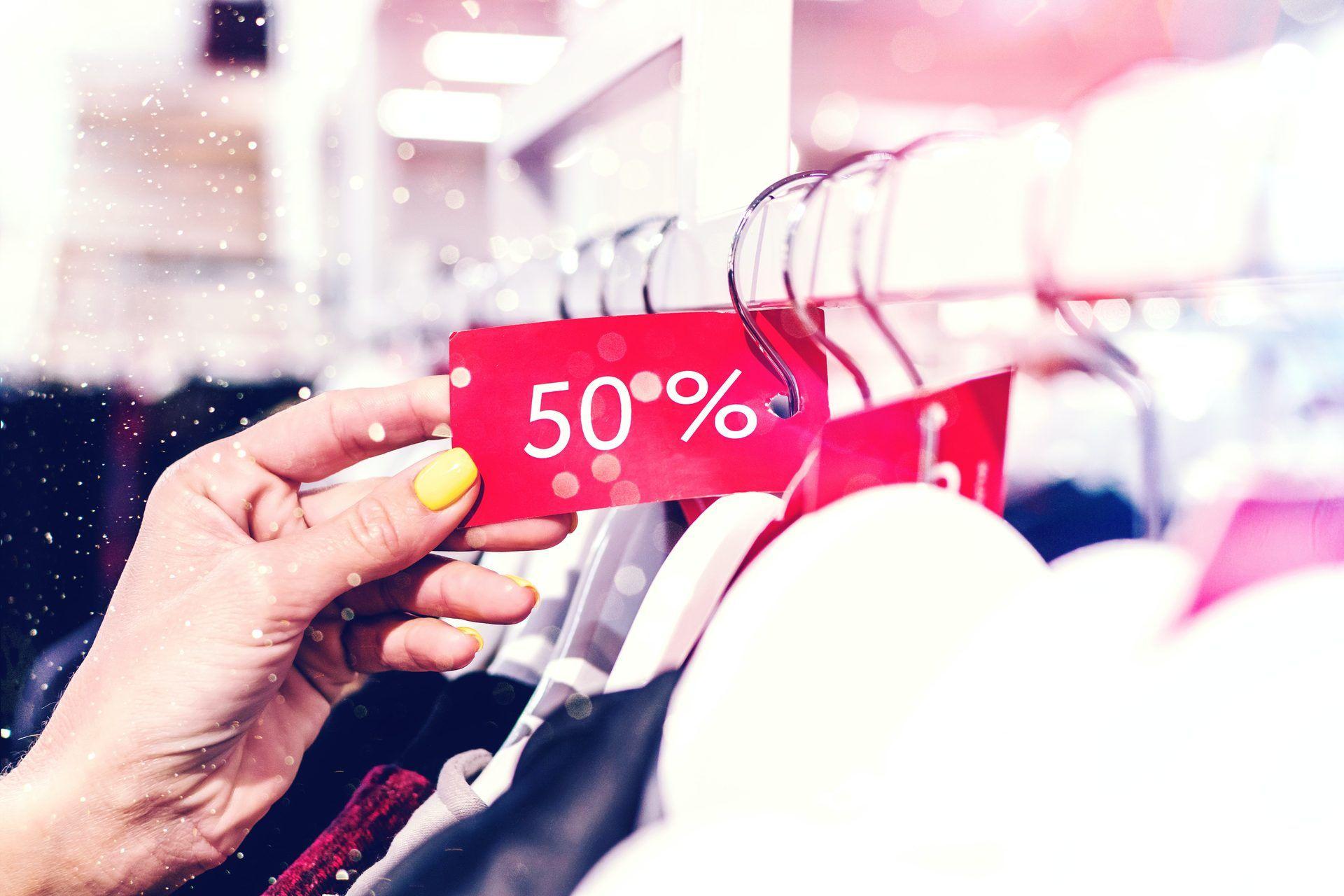
Demand prediction
A company needs to estimate demand if it wants to give its clients a truly tailored experience. The corporation will profit from machine learning’s improved inventory planning, ensuring the product is stocked following demand forecasts.
Customer segmentation
Customer segmentation in the retail and e-commerce industries refers to leveraging past customer data to separate customers into groups based on similar behavior and interests. Based on characteristics like gender, age, geography, buying habits, etc., segmentation can be done successfully with machine learning.
This gives businesses a better understanding of the customers in each segment and enables them to provide customized marketing services. Customers respond more favorably when they believe the company is meeting their demands. Long-term client retention and happiness, which result in revenue growth, are among the key advantages of customer segmentation for online retail businesses.
Logistics support (Supply chain management)
Machine learning algorithms rely on the same data to choose the items’ most effective delivery routes. Retailers may simultaneously improve customer experience by delivering goods faster and saving money if they use smart technology to boost logistical planning.
Additionally, systems can account for the requirement to lower harmful air emissions from road transportation.
Personalized offers
The best choices regarding what kind of goods will suit the user and at which point he/she will need them are then made based on this information and other information, using the user’s behavior, including his/her recent purchases, Google search history, social network remarks, and solvency.
Predictive analytics
Predictive analytics has evolved into a potent tool for previously unattainable merchants. They are now based on common sense and a large array of historical, current, and supposed data sources due to machine learning and artificial intelligence.
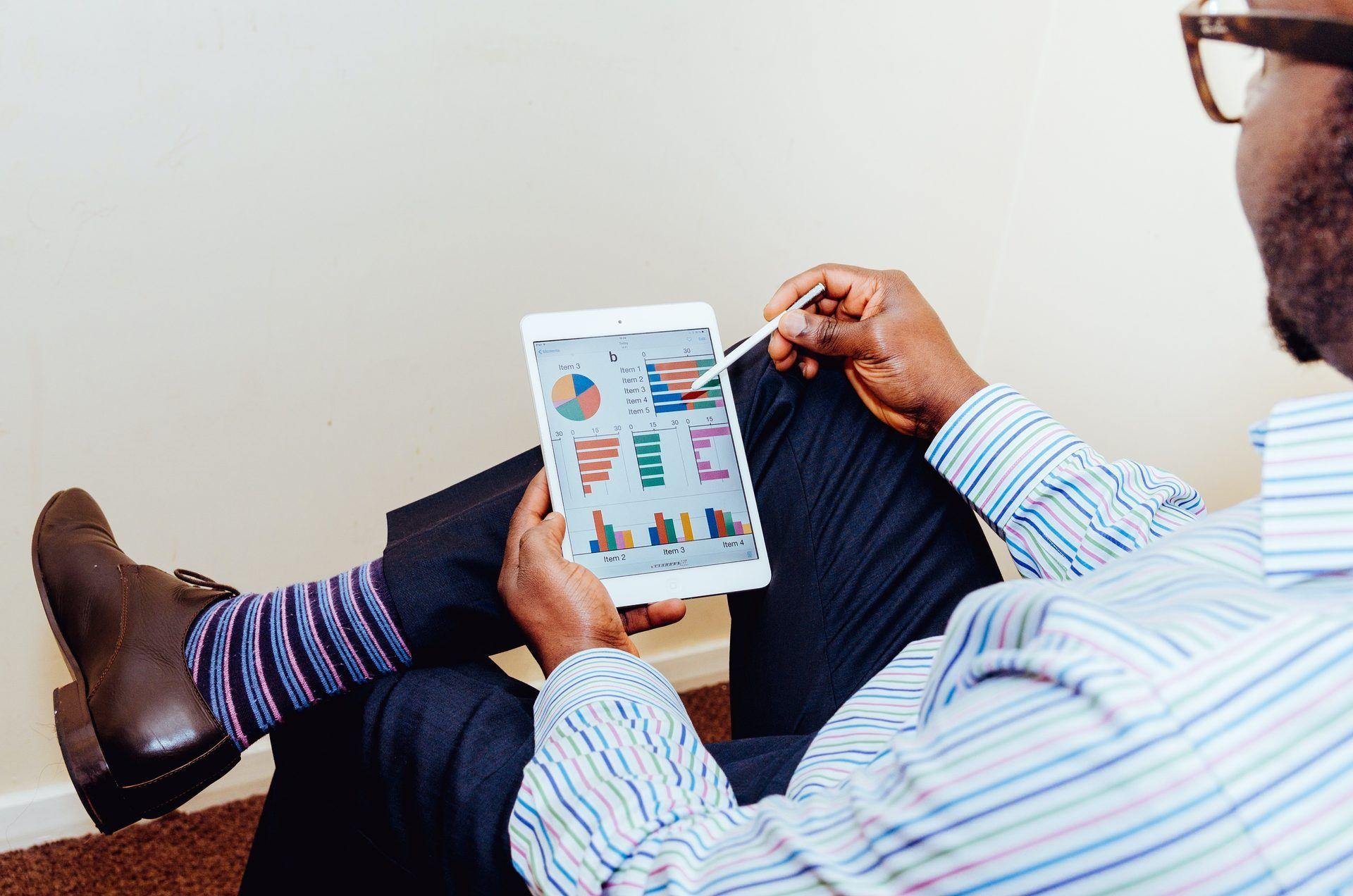
Is artificial intelligence better than human intelligence? Check out the cons of artificial intelligence
Market basket analysis
Analyzing and looking for connections between various entities that frequently occur together is the technique of market basket analysis. It is a method for analyzing buying trends predicated on the notion that when clients buy one thing, they will also buy other similar products.
For instance, if a consumer buys milk, he may also buy sugar, tea, or coffee. ML technologies easily process enormous data and find the right outcome.
Churn rate prediction
Five times more money is spent on gaining a new client than keeping an existing one. With machine learning, a business may spot circumstances likely to cause a customer to leave so that the most urgent measures can be taken to keep him on board.
Location optimization
Businesses can use the technology to target customers depending on their location and figure out quicker and more effective ways to deliver items to customers.
Classification of customer reviews
Any e-commerce website must prioritize customer product reviews. These reviews support data organizations’ efforts to understand consumer experiences, conduct market research, monitor product reputation, and evaluate public opinion. A machine learning model that predicts whether a customer would recommend a product to others based on their review is possible.
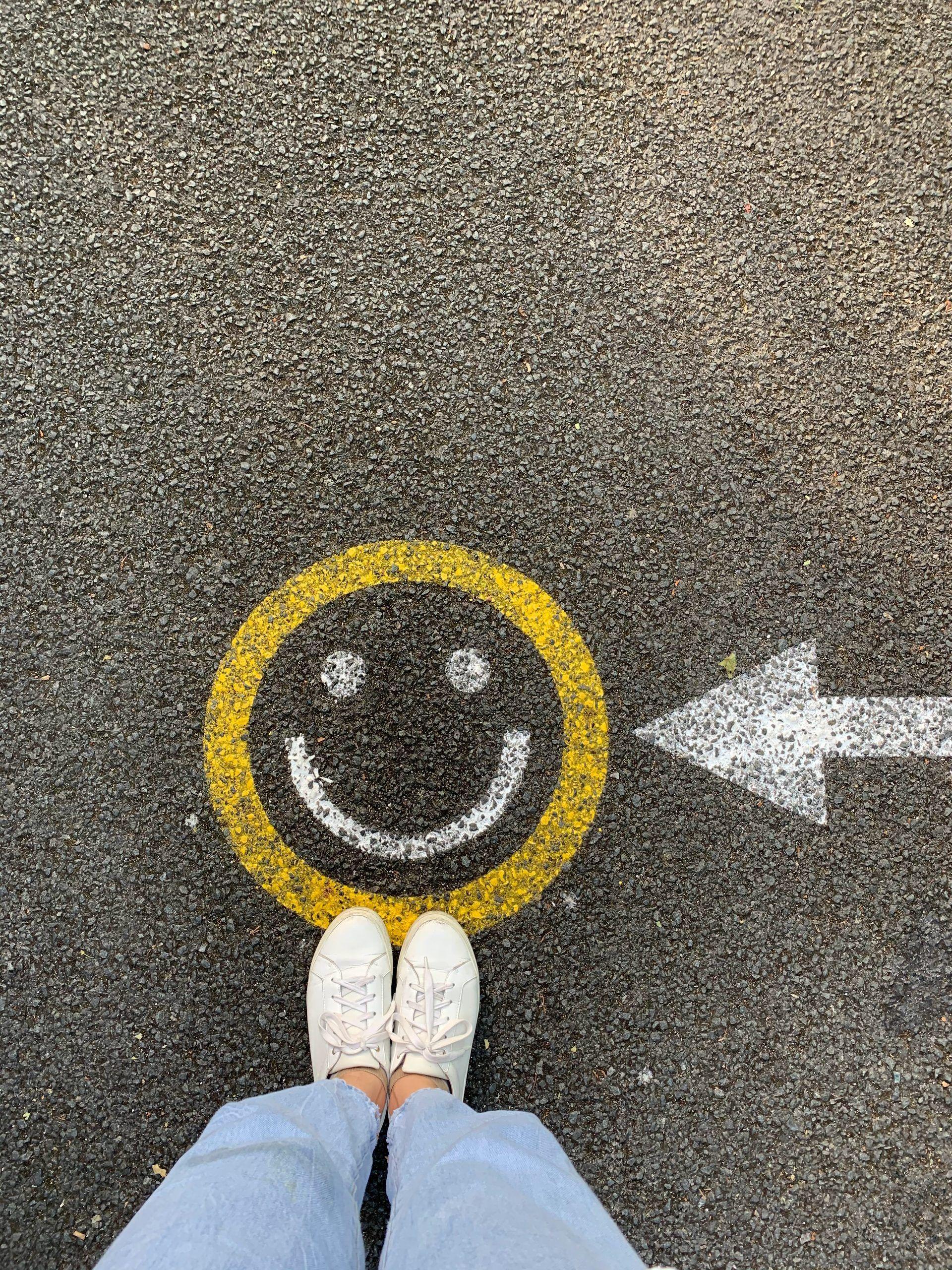
Before making an online purchase, 81% of shoppers seek research online.
Fraud detection
Machine learning and AI successfully detect and prevent credit card fraud when making purchases in-person or online because the system can learn for itself.
In addition to having unrestricted access to data, machine learning algorithms may also be able to stop fraudulent coupon and discount activities by monitoring user activity coming from a particular IP address.
Determining customer lifetime value (CLTV)
The amount they spend on the offers, their consistency, their payment history, and the number of times they’ve purchased can all be used to assess which consumers have high lifetime values.
Businesses can improve their marketing campaigns with the use of this information. It would thus enhance their proportion of the most valuable clients, resulting in a consistent flow of income.
Document work automation
Machine learning may also be used to examine internal data from businesses, such as how it handles human resources.
It enables you as a store to give your staff members more flexibility, free them up from mundane jobs, and better arrange their workdays so they can stay engaged, productive, and focused on providing excellent customer service.
Cross-sell prediction
You can create a model that predicts whether a customer needs a specific product or service that should be cross-sold.
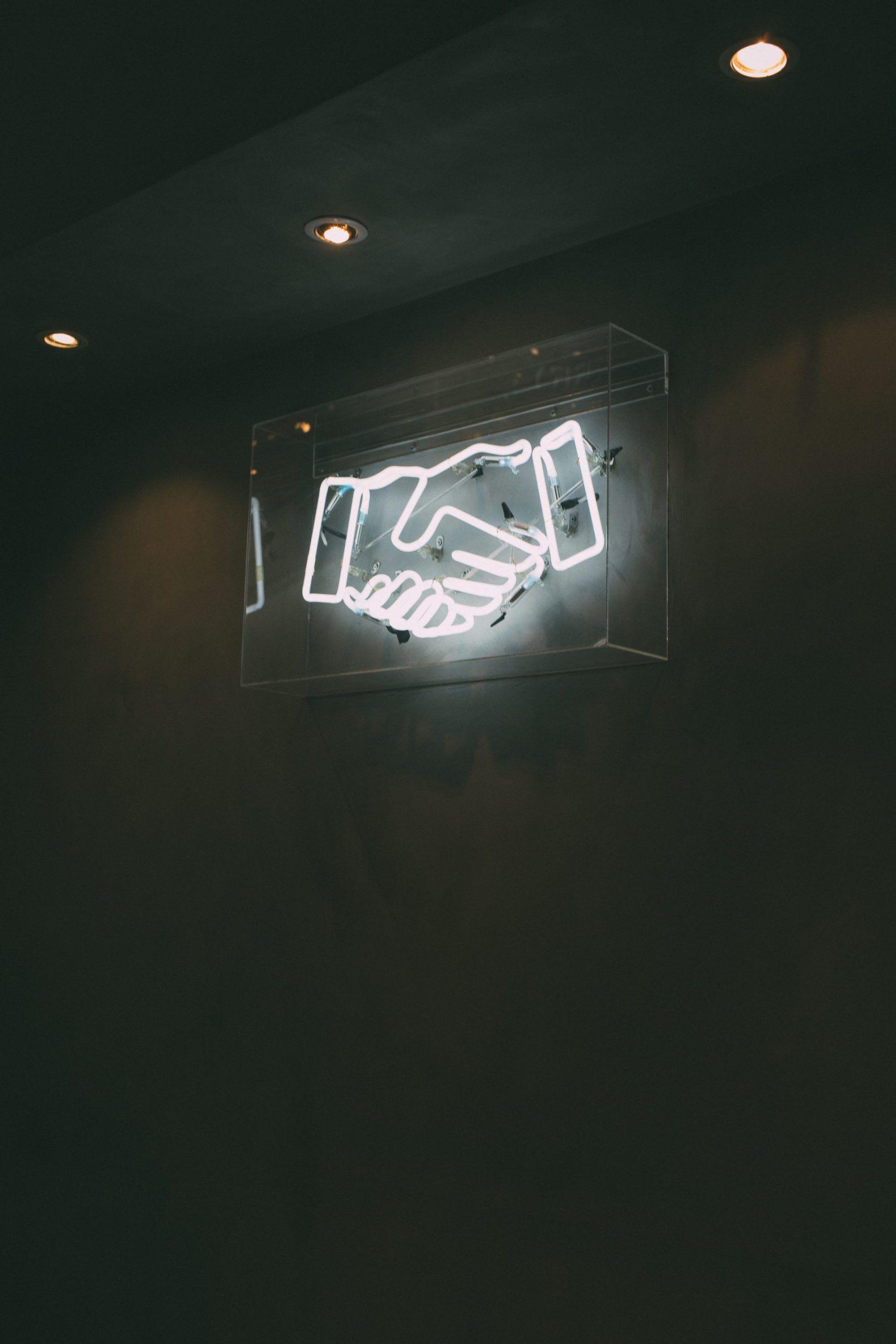
Selling a separate good or service to customers to boost the value of a transaction is known as cross-selling. Along with the original product that a consumer owns, cross-selling refers to goods or services that meet their complementary demands.
Merchandising
Machine learning may ensure that an online client has the same visual merchandising experience as an offline customer. Customers have claimed the importance of product photos in the sales process. Businesses now employ machine learning to provide clients with visual effects.
Real-life examples of machine learning in retail
How brands are using machine learning in retail? Let’s find out. These brands are already begun to use machine learning in retail:
- Amazon
- Sephora
- Netflix
- H&M
- Apotek Hjärtat
- North Face
- Costco
It’s time to take a closer look at how they implement ML algorithms in their business operations:
Staff-less stores: Amazon
Amazon, the largest online retailer in the world, launched its first public store without any employees at the beginning of 2018. (previously, for two years, the store without cashiers and staff was available only to company employees).
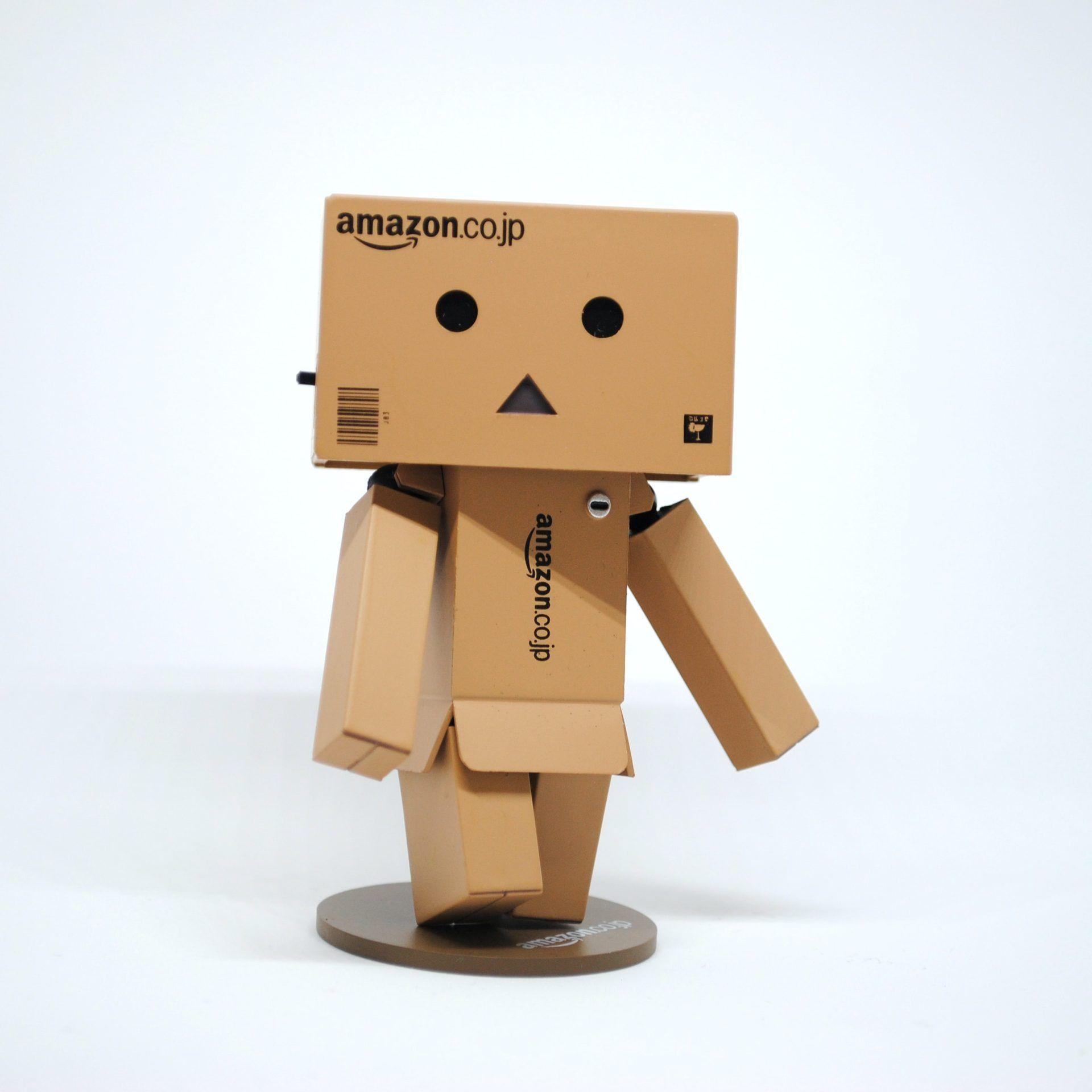
It is not shocking that Amazon chose to experiment with the most recent AI advancements outside of the automated payment system.
E-commerce machine learning projects: Sephora
By developing a machine learning application that allows retail consumers to select specific hues by merely uploading a photo, Sephora is pushing the boundaries of innovation in online commerce.
The platform is anticipated to have significant effects outside of the cosmetics sector. The customer will be able to perceive the product’s benefits after the purchase thanks to this technology, which will be an organic addition to Sephora’s online shopping experience and promote transactions.
Demand prediction: Netflix
Since its start, Netflix has been delivering the material viewers want by leveraging big data and machine learning to understand how its consumers consume television and cinema content.
Their “% match” rating is the most recent example of how they provide this type of data-based recommendation engine. This data has informed tactical decisions such as how they release full seasons all at once, auto-play the next episode, and offer recommendations for how likely you are to enjoy a related film or show. All of the original content they create has also been influenced by these facts.
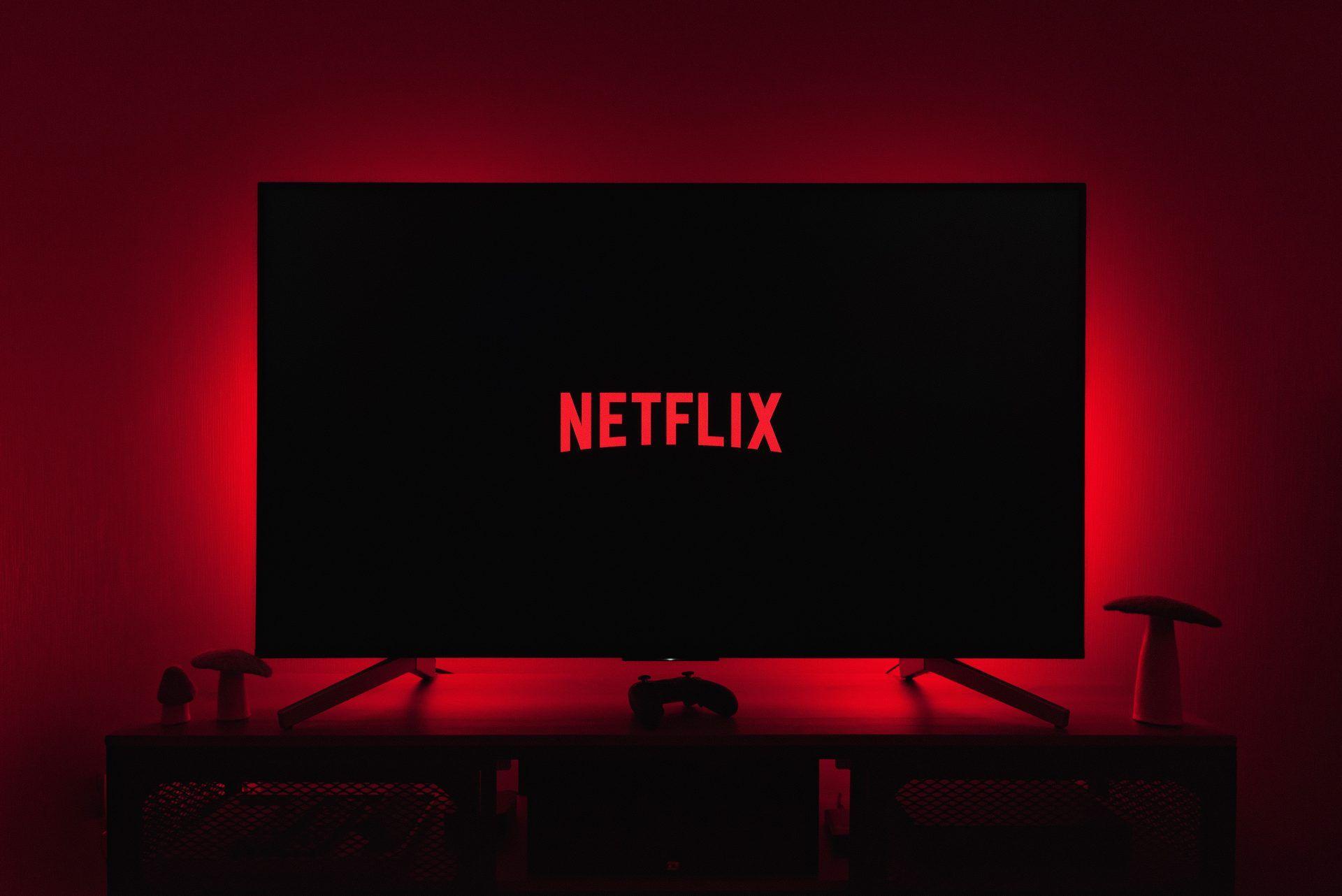
Netflix executives estimate that machine learning insights help them save $1 billion annually.
Machine learning vs data science: What are the differences?
Retail demand forecasting machine learning: H&M
The Swedish H&M division started utilizing machine learning to choose various locations in 2018. Through this strategy, the company intends to make up for the worst sales in its 71-year history, which have seen a decline in sales for 10 straight quarters.
Analysts are doubtful about H&M’s plan. However, the case of one H&M store in a posh area of Stockholm shows that machine learning can be extremely beneficial.
Retail pricing machine learning: Apotek Hjärtat
The largest private pharmacy chain in Sweden, Apotek Hjärtat, with about 3000 employees and roughly 390 pharmacies. They own pharmacies throughout Sweden in major towns, rural areas, and inhabited places.
The business has enhanced its approach through AI by offering more accurate pricing compared to rivals in both online and offline stores.
Machine learning projects for marketing: North Face
North Face has been utilizing AI and machine learning to provide website visitors with a highly personalized purchasing experience called “Shop with IBM Watson.”
Customers can talk directly into their phones after downloading the app to use IBM Watson, an AI system. The virtual assistant guides users through a series of questions and learns from your responses to present you with the most pertinent products for your preferences and needs, like a human salesperson who may help you make the best choice.
Machine learning in supermarkets: Costco
Costco employs machine learning to sustain the productivity and viability of its fresh food section. Costco produced more fresh food than was required since all of its unsold or broken goods were given.
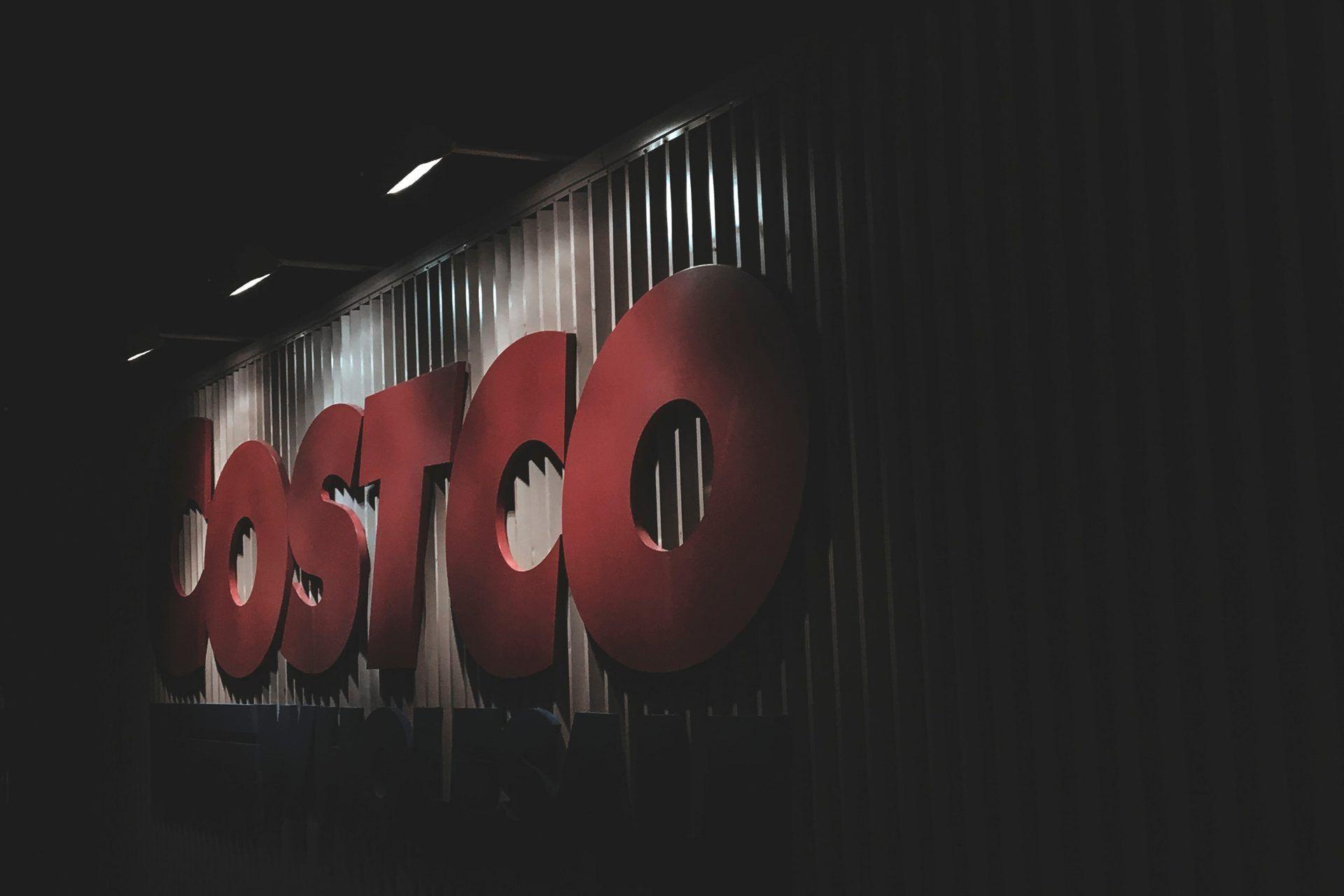
Check out the 15 real-life examples of machine learning
Prospects for machine learning in retail
More than 70% of retail and consumer product companies will employ intelligent automation solutions along their supply chains, predicts an IBM survey.
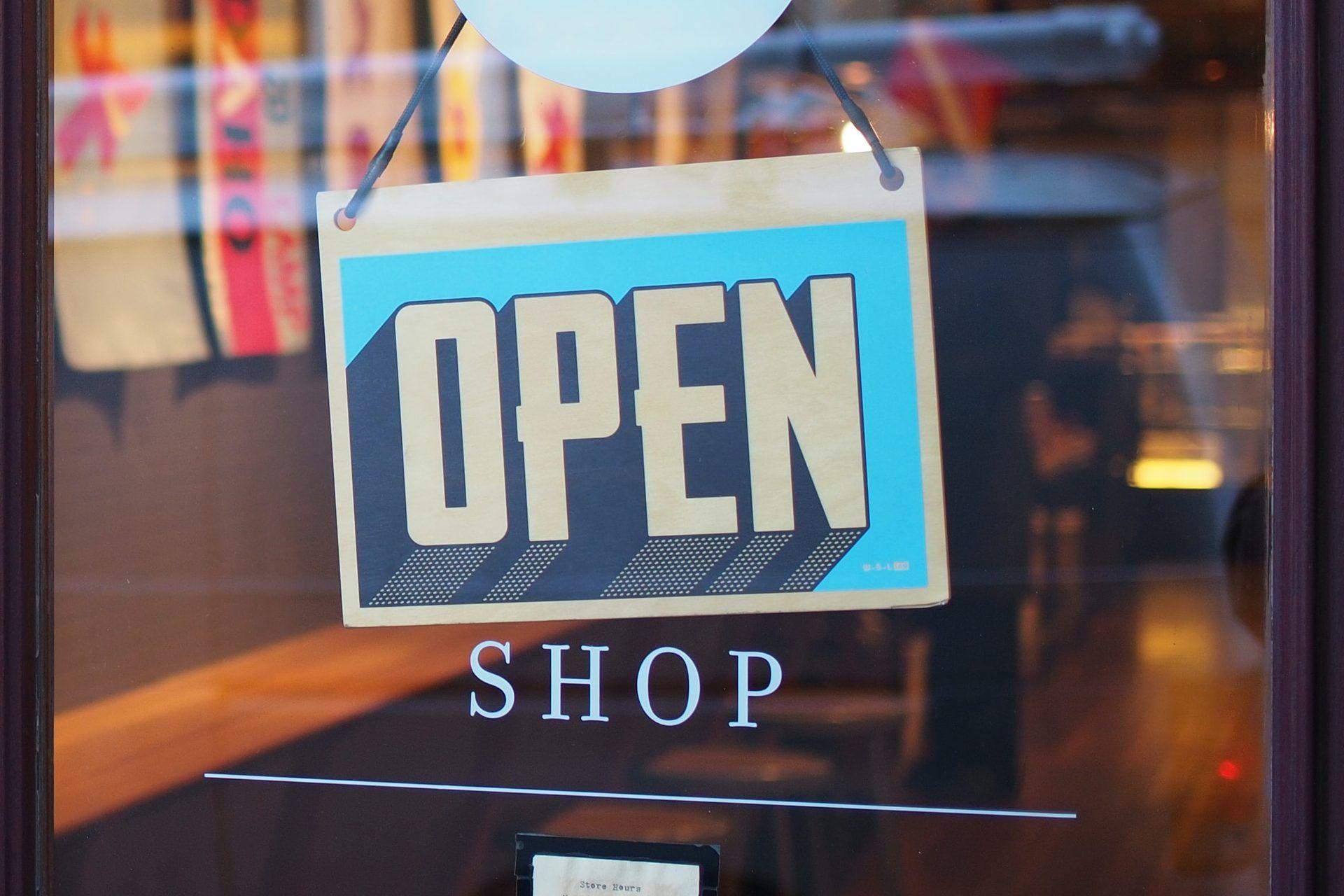
Retailers plan to use AI in the following 6 areas:
- Supply Chain Planning (85%)
- Demand forecasting (85%)
- Customer Intelligence (79%)
- Marketing and advertising (75%)
- Warehouse operations (73%)
- Pricing and promotion (73%)
Check out the 7 opportunities for retailers from data management
Conclusion
Most successful firms now rely on ML solutions, which breathe new life into outdated predictive analytics techniques and transform them into highly effective business insight and prediction tools. These applications are advantageous in various sectors, including marketing, e-commerce, and retail. It turns into the engine that propels a business’s decisions and forecasts its future success.
In a consumer paradigm that is always changing, the retail business is expanding and facing new challenges. Using cutting-edge technology to provide these necessities can make the difference between success and obsolescence.