- Physicists have employed artificial intelligence to simplify a difficult quantum problem that previously required 100,000 equations to a reasonable effort of as few as four equations—all while maintaining accuracy.
- The discoveries, which were published in Physical Review Letters on September 23rd, have the potential to transform how scientists study systems containing many interacting electrons.
- Furthermore, if the approach can be applied to different scenarios, it may aid in the development of materials with desirable properties such as superconductivity or usefulness for clean energy generation.
Physicists have used artificial intelligence to reduce a challenging quantum issue that formerly needed 100,000 equations to a manageable effort of as few as four equations—all without losing accuracy.
Benefits of the new artificial intelligence
The findings, which were published in the September 23 issue of Physical Review Letters, have the potential to change how scientists analyze systems with multiple interacting electrons. Furthermore, if the technique is adaptable to other situations, it might possibly help in the creation of materials with desirable features such as superconductivity or utility for clean energy generation.
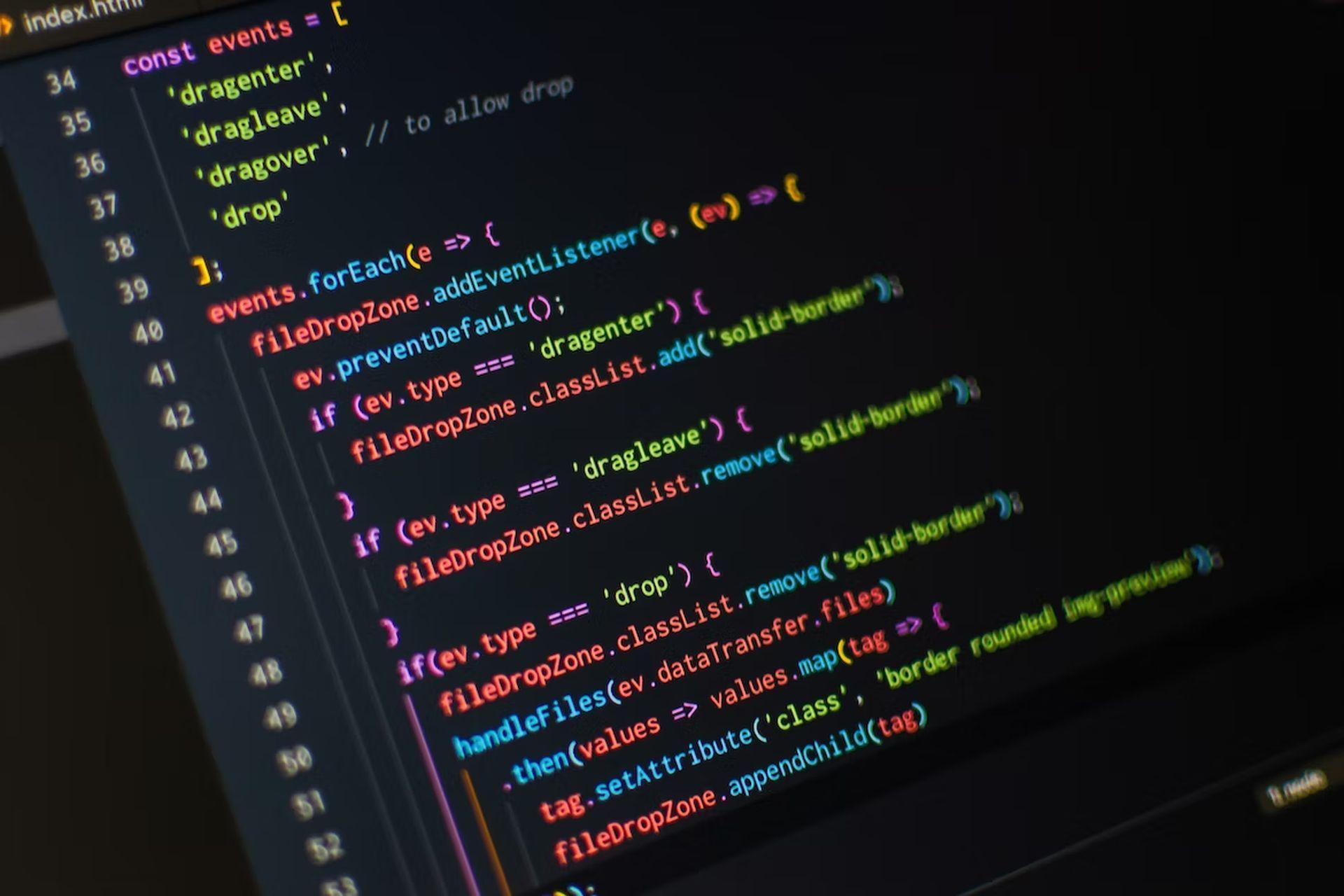
Domenico Di Sante, a visiting research fellow at the Flatiron Institute’s Center for Computational Quantum Physics (CCQ) in New York City and an assistant professor at the University of Bologna in Italy, and the study’s primary author said, “We start with this huge object of all these coupled-together differential equations; then we’re using machine learning to turn it into something so small you can count it on your fingers.”
The difficult problem that artificial intelligence has simplified concerns how electrons flow on a gridlike lattice. When two electrons occupy the same lattice position, they interact. This configuration, known as the Hubbard model, idealizes numerous major types of materials and allows scientists to study how electron behavior gives birth to desired phases of matter, such as superconductivity, in which electrons flow through a material without resistance. The model also acts as a testing ground for new approaches before they are applied to more sophisticated quantum systems.
However, the Hubbard model is deceptively simple. Even a small number of electrons and cutting-edge computational tools are insufficient to solve the problem. Because electrons’ outcomes can get quantum mechanically entangled as they interact.
Even when separated by a large distance on distinct lattice sites, the two electrons cannot be addressed separately, hence physicists must deal with all of the electrons at once rather than one at a time. More electrons result in more entanglements, making the computing job progressively more difficult.
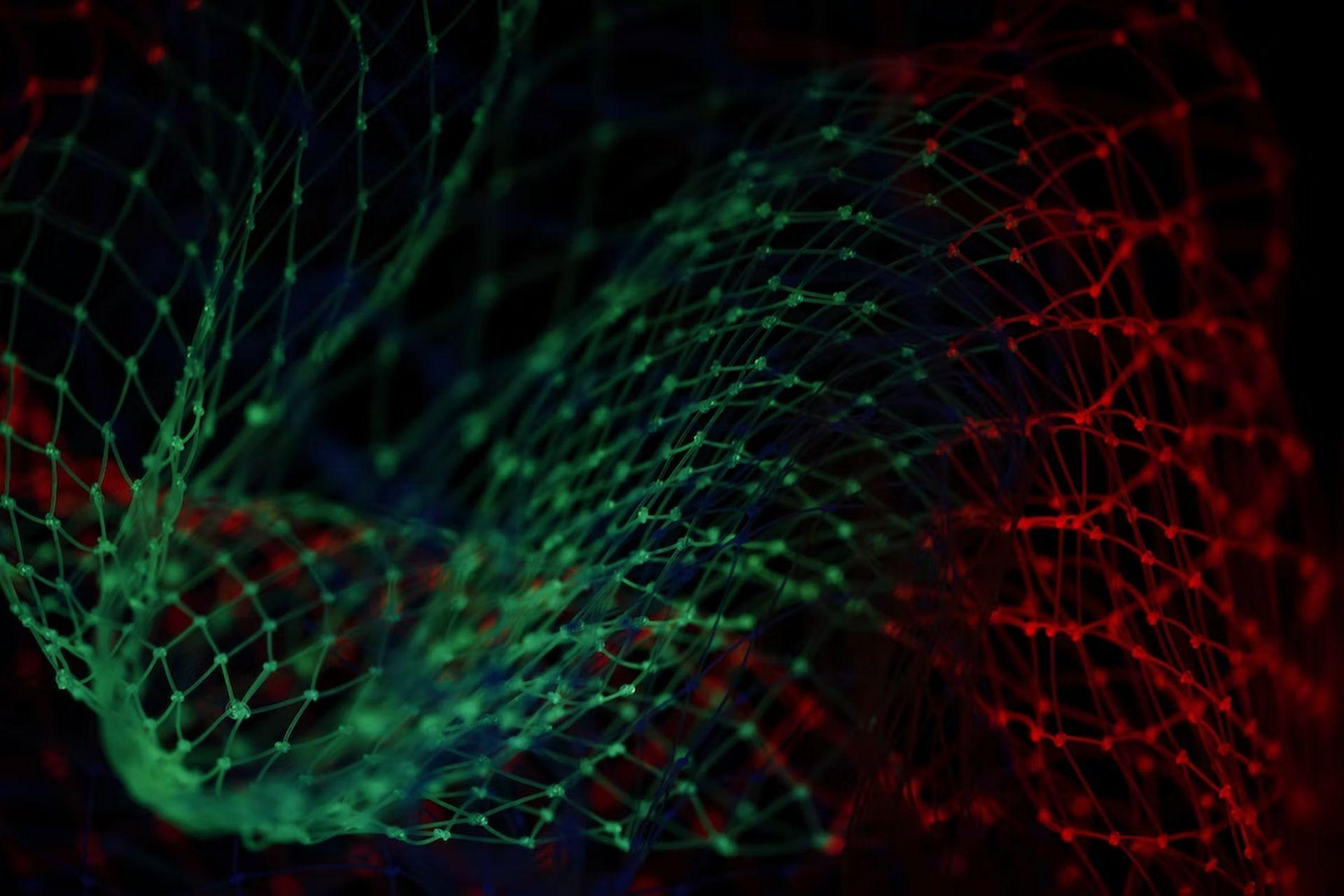
A renormalization group is one technique for analyzing a quantum system. This is a mathematical instrument used by physicists to examine how the behavior of a system, such as the Hubbard model, varies as scientists adjust characteristics such as temperature or examine the attributes on different scales.
Unfortunately, a renormalization group that keeps track of all potential electron couplings and makes no sacrifices might have tens of thousands, hundreds of thousands, or even millions of unique equations that must be solved. Furthermore, the equations are difficult to understand: each one describes the interaction of two electrons.
Working principle of the artificial intelligence
Di Sante and his colleagues wondered whether they might utilize a neural network, a machine learning technology, to make the renormalization group more controllable. The neural network resembles a frenzied switchboard operator crossed with survival-of-the-fittest evolution. The machine learning program begins by making connections inside the full-size renormalization group.
The rise of artificial intelligence customer services
The neural network then adjusts the strength of those connections until it discovers a tiny set of equations that produces the same answer as the original, jumbo-sized renormalization group. Even with only four equations, the program’s output captured the physics of the Hubbard model.
Di Sante stated, “It’s essentially a machine that has the power to discover hidden patterns. When we saw the result, we said, ‘Wow, this is more than what we expected.’ We were really able to capture the relevant physics.”
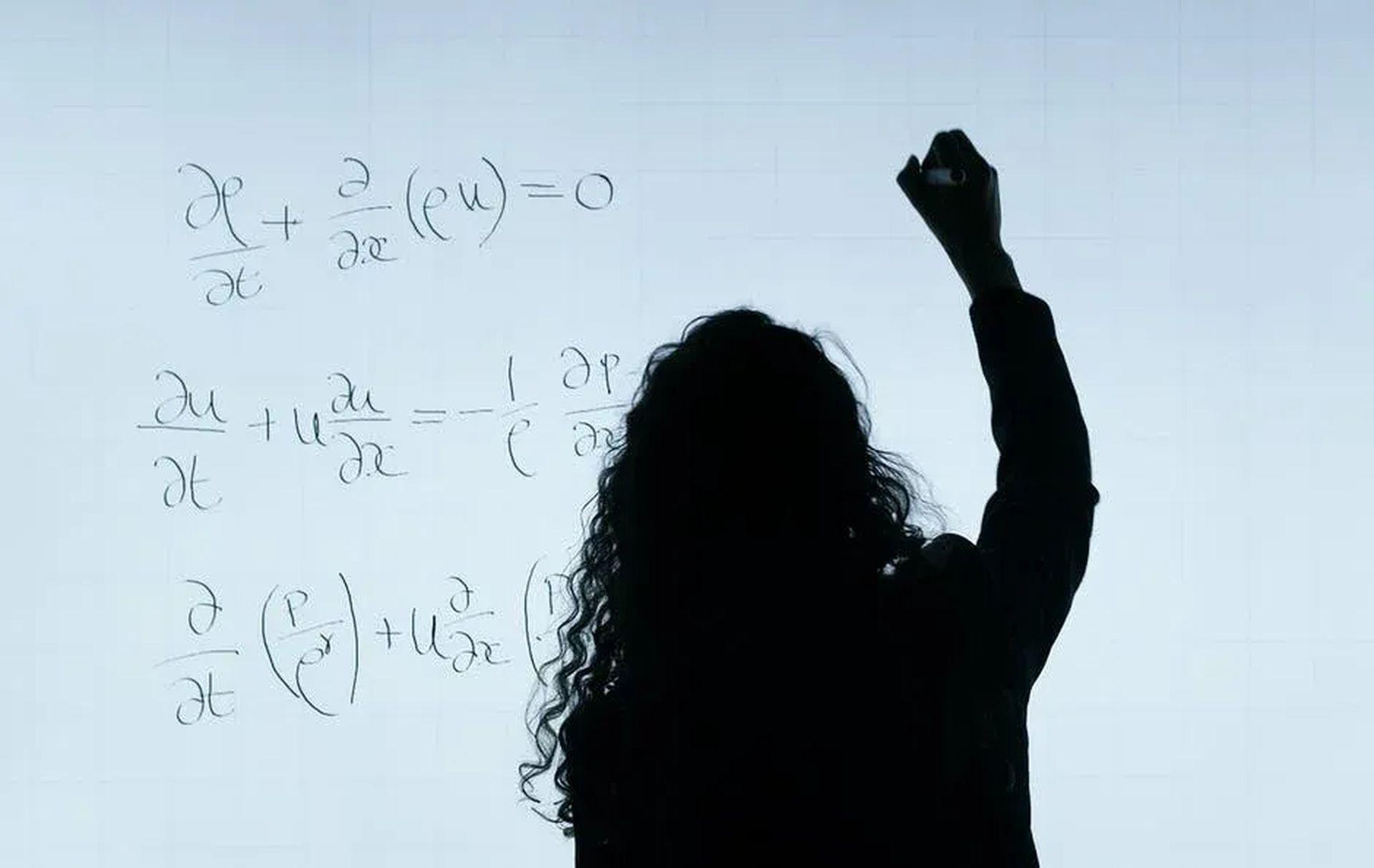
The machine learning software’s training took a lot of computing power, and the program ran for weeks. The good news, according to Di Sante, is that now that their software has been taught, they can adjust it to work on different problems without having to start from zero.
He and his colleagues are also looking into what machine learning is “learning” about the system, which might yield new insights that would otherwise be difficult for physicists to comprehend.
France starts using artificial intelligence to discover taxable swimming pools
The largest unknown is how well the new method works on more complicated quantum systems, such as materials in which electrons interact across vast distances. Furthermore, Di Sante believes that there is intriguing potential for employing artificial intelligence in other domains that deal with renormalization groups, such as cosmology and neurology.