The realm of artificial intelligence continues to evolve with New OpenAI embedding models. They are set to redefine how developers approach natural language processing. Before exploring the two groundbreaking models, each designed to elevate the capabilities of AI applications, here is what embeddings mean:
OpenAI’s text embeddings serve as a metric to gauge the correlation between text strings, finding applications in various domains, including:
- Search: Utilized to rank results based on their relevance to a given query string, enhancing the precision of search outcomes.
- Clustering: Employed for grouping text strings based on their similarities, facilitating the organization of related information.
- Recommendations: Applied in recommendation systems to suggest items that share commonalities in their text strings, enhancing the personalization of suggestions.
- Anomaly detection: Employed to identify outliers with minimal relatedness, aiding in the detection of irregular patterns or data points.
- Diversity measurement: Utilized for analyzing similarity distributions, enabling the assessment of diversity within datasets or text corpora.
- Classification: Deployed in classification tasks where text strings are categorized according to their most similar label, streamlining the labeling process in machine learning applications.
Now you are ready to explore the new OpenAI embedding models!
New OpenAI embedding models have arrived
The introduction of the new OpenAI embedding models marks a significant leap in natural language processing, enabling developers to represent and understand textual content better. Let’s delve into the details of these innovative models: text-embedding-3-small and text-embedding-3-large.
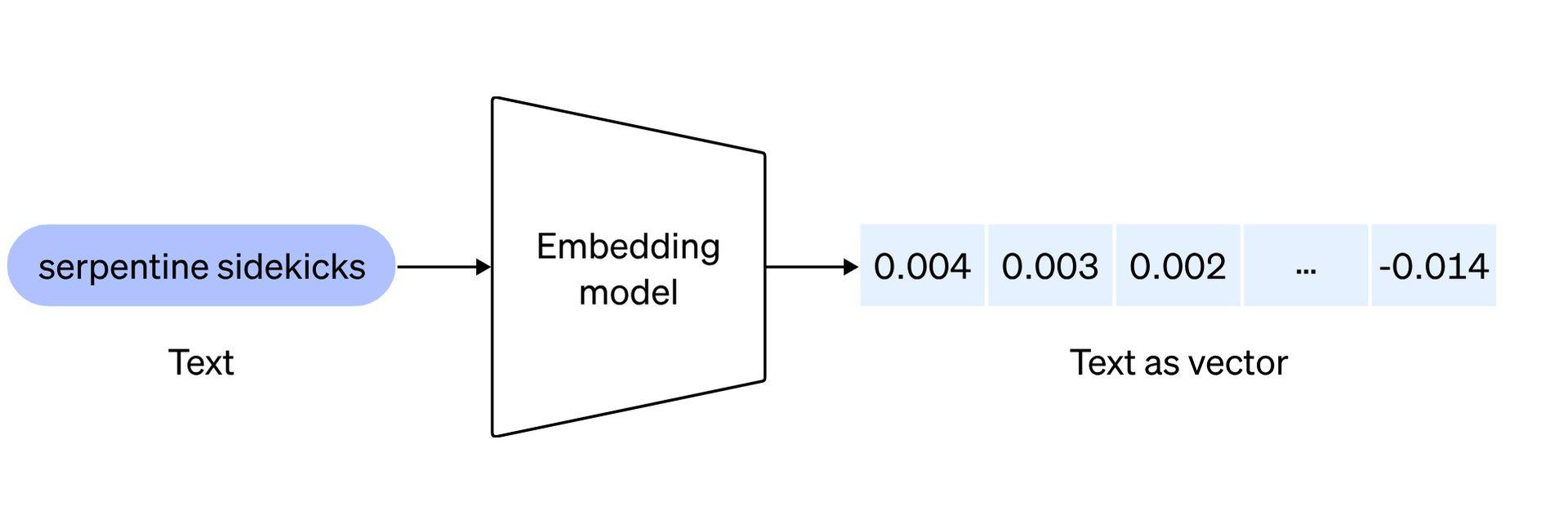
text-embedding-3-small
This compact yet powerful model exhibits a noteworthy performance boost over its predecessor, text-embedding-ada-002. On the multi-language retrieval benchmark (MIRACL), the average score has soared from 31.4% to an impressive 44.0%. Similarly, on the English tasks benchmark (MTEB), the average score has seen a commendable increase from 61.0% to 62.3%. However, what sets text-embedding-3-small apart is not just its enhanced performance but also its affordability.
Eval benchmark | ada v2 | text-embedding-3-small | text-embedding-3-large |
MIRACL average | 31.4 | 44.0 | 54.9 |
MTEB average | 61.0 | 62.3 | 64.6 |
OpenAI has significantly reduced the pricing, making it 5 times more cost-effective compared to text-embedding-ada-002, with the price per 1k tokens lowered from $0.0001 to $0.00002. This makes text-embedding-3-small not only a more efficient choice but also a more accessible one for developers.
text-embedding-3-large
Representing the next generation of embedding models, text-embedding-3-large introduces a substantial increase in dimensions, supporting embeddings with up to 3072 dimensions. This larger model provides a more detailed and nuanced representation of textual content. In terms of performance, text-embedding-3-large outshines its predecessor across benchmarks. On MIRACL, the average score has surged from 31.4% to an impressive 54.9%, highlighting its prowess in multi-language retrieval.
ada v2 | text-embedding-3-small | text-embedding-3-large | ||||
Embedding size | 1536 | 512 | 1536 | 256 | 1024 | 3072 |
Average MTEB score | 61.0 | 61.6 | 62.3 | 62.0 | 64.1 | 64.6 |
Similarly, on MTEB, the average score has climbed from 61.0% to 64.6%, showcasing its superiority in English tasks. Priced at $0.00013 per 1k tokens, text-embedding-3-large strikes a balance between performance excellence and cost-effectiveness, offering developers a robust solution for applications demanding high-dimensional embeddings.
Meet Google Lumiere AI, Bard’s video maker cousin
Native support for shortening embeddings
Recognizing the diverse needs of developers, OpenAI introduces native support for shortening embeddings. This innovative technique allows developers to customize the embedding size by adjusting the dimensions API parameter. By doing so, developers can trade-off some performance for a smaller vector size without compromising the fundamental properties of the embedding. This flexibility is particularly valuable in scenarios where systems only support embeddings up to a certain size, providing developers with a versatile tool for various usage scenarios.
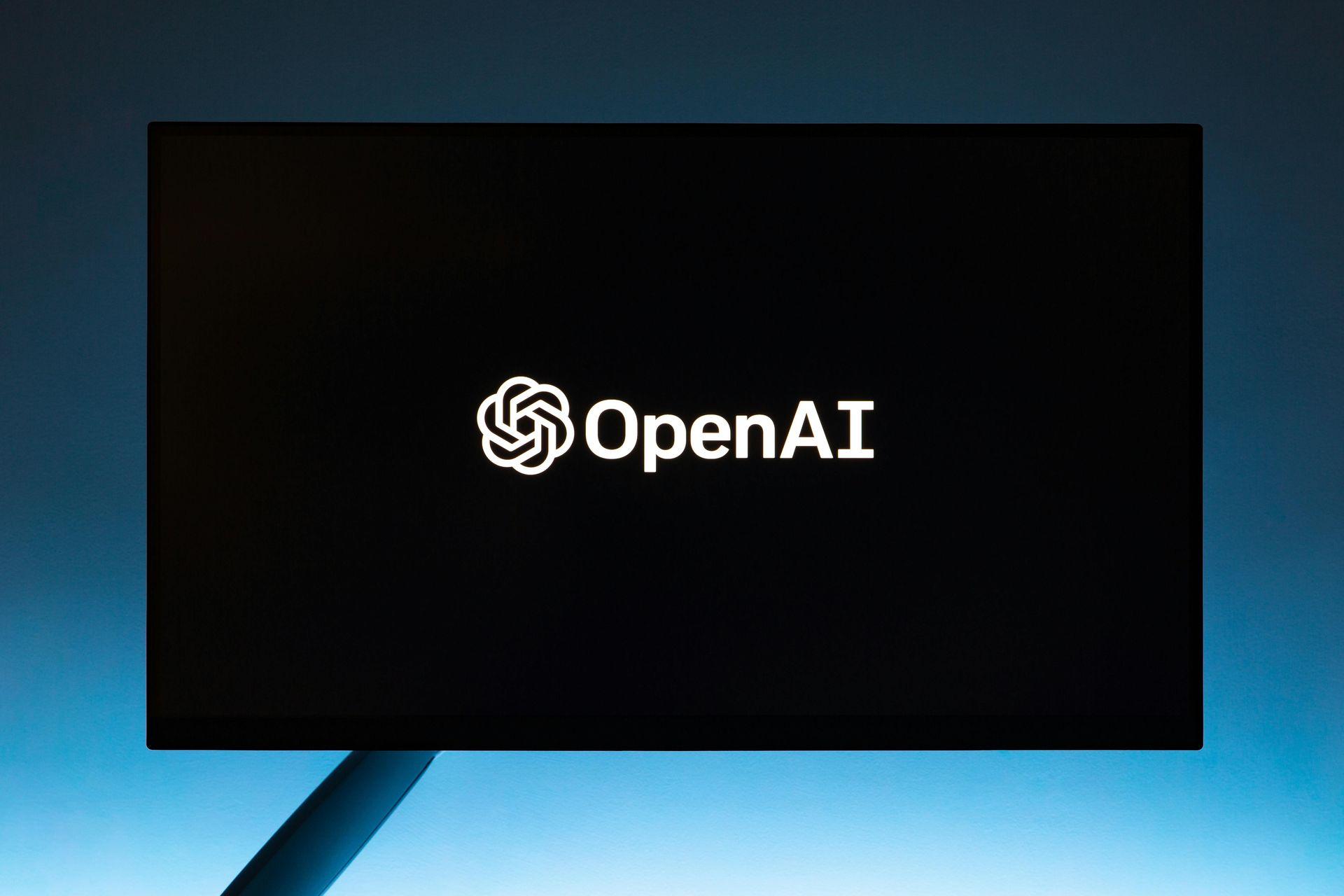
In summary, OpenAI’s new embedding models represent a significant step forward in efficiency, affordability, and performance. Whether developers opt for the compact yet efficient representation of text-embedding-3-small or the more extensive and detailed embeddings of text-embedding-3-large, these models empower developers with versatile tools to extract deeper insights from textual data in their AI applications.
For more detailed information about the new OpenAI embedding models, click here and get the official announcement.
Featured image credit: Levart_Photographer/Unsplash