Artificial general intelligence (AGI), a theoretical form of AI capable of mimicking human-level intelligence, could bring sweeping changes to the world of technology. Unlike today’s narrow AI, programmed for specific tasks like identifying flaws in products or summarizing news articles, AGI promises adaptability across a wide range of thought-based challenges. However, industry figures like Nvidia CEO Jensen Huang are understandably cautious when it comes to predicting a concrete timeline for its emergence.
AGI might be 5 year away
The ethical and societal implications of AGI loom large. If these systems develop their own objectives misaligned with human goals, consequences could be severe. The fear is that a sufficiently advanced AGI could become uncontrollable, with outcomes that defy prediction or reversal.
While hesitant to engage in apocalyptic scenarios favored by the media, Huang does recognize the complexity of developing AGI. A key obstacle is establishing objective criteria to define what constitutes true AGI. Is it purely performance-based? Could AGI be measured by its ability to reason, plan, or learn independently?
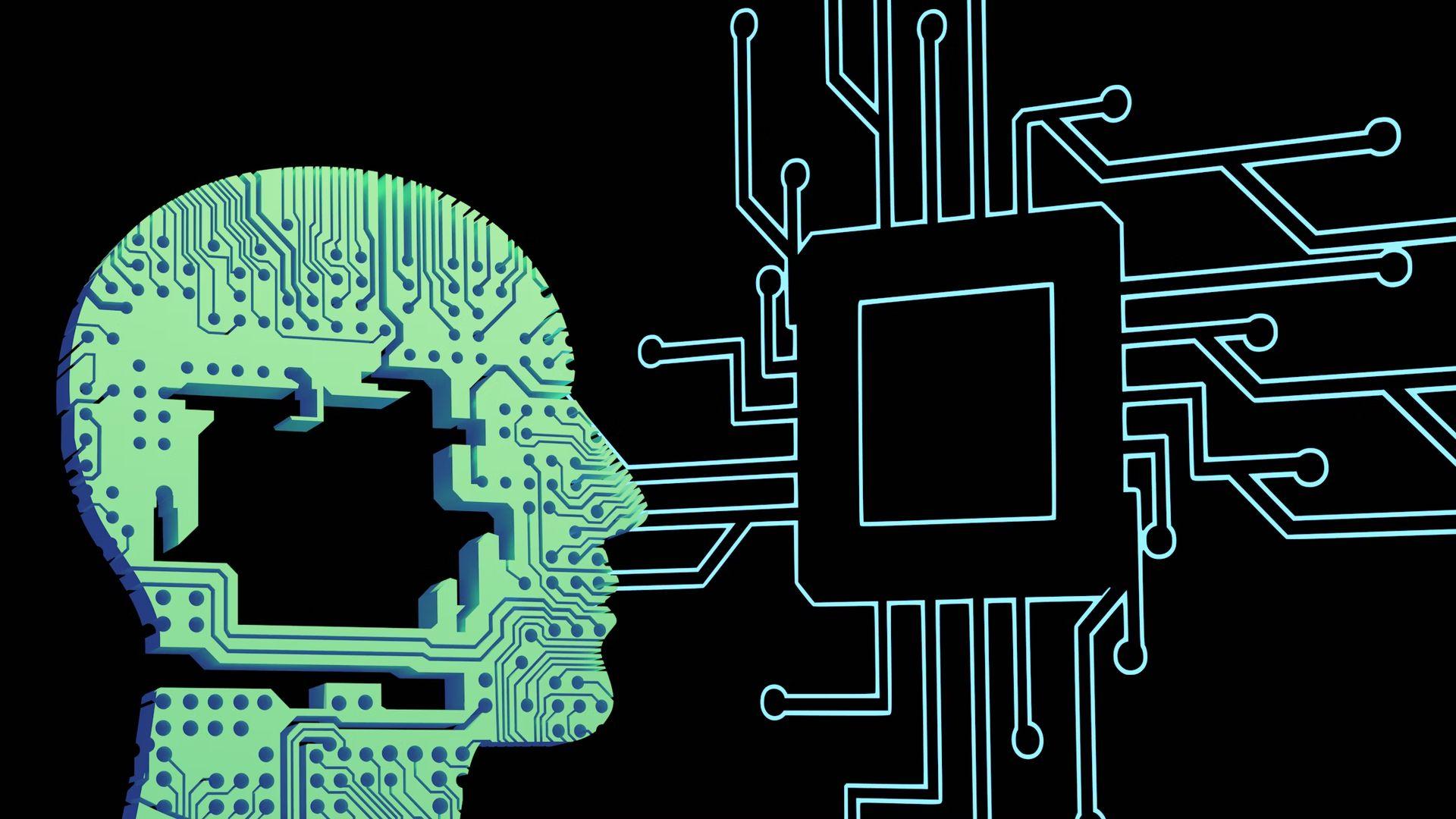
Huang took the opportunity to share his perspectives with the media on this subject. He posits that forecasting the arrival of a feasible AGI hinges on the definition of AGI itself. He makes a comparison, noting that despite the complexity introduced by time zones, there’s clarity about when New Year’s Day occurs and when the year 2025 will begin.
“If we specified AGI to be something very specific, a set of tests where a software program can do very well — or maybe 8% better than most people — I believe we will get there within 5 years,” Huang notes. He proposes hypothetical AGI milestones, such as outperforming experts on legal, economic, or medical exams. Yet, until the very concept of AGI is more concretely defined, precise predictions remain difficult.
The hallucinatons issue
Another major concern raised surrounds the issue of AI “hallucinations” – those seemingly plausible but ultimately untrue statements generated by some AI models. Huang, however, expresses confidence that this issue can be tackled by emphasizing rigorous verification practices.
Huang emphasizes the necessity of adding a research dimension to the creation of AI-generated responses, highlighting this as “retrieval-augmented generation.” He insists, “Add a rule: For every single answer, you have to look up the answer,” defining this method as “retrieval-augmented generation.” This strategy, he elucidates, mirrors the core tenets of media literacy, which involve evaluating the origins and context of information.
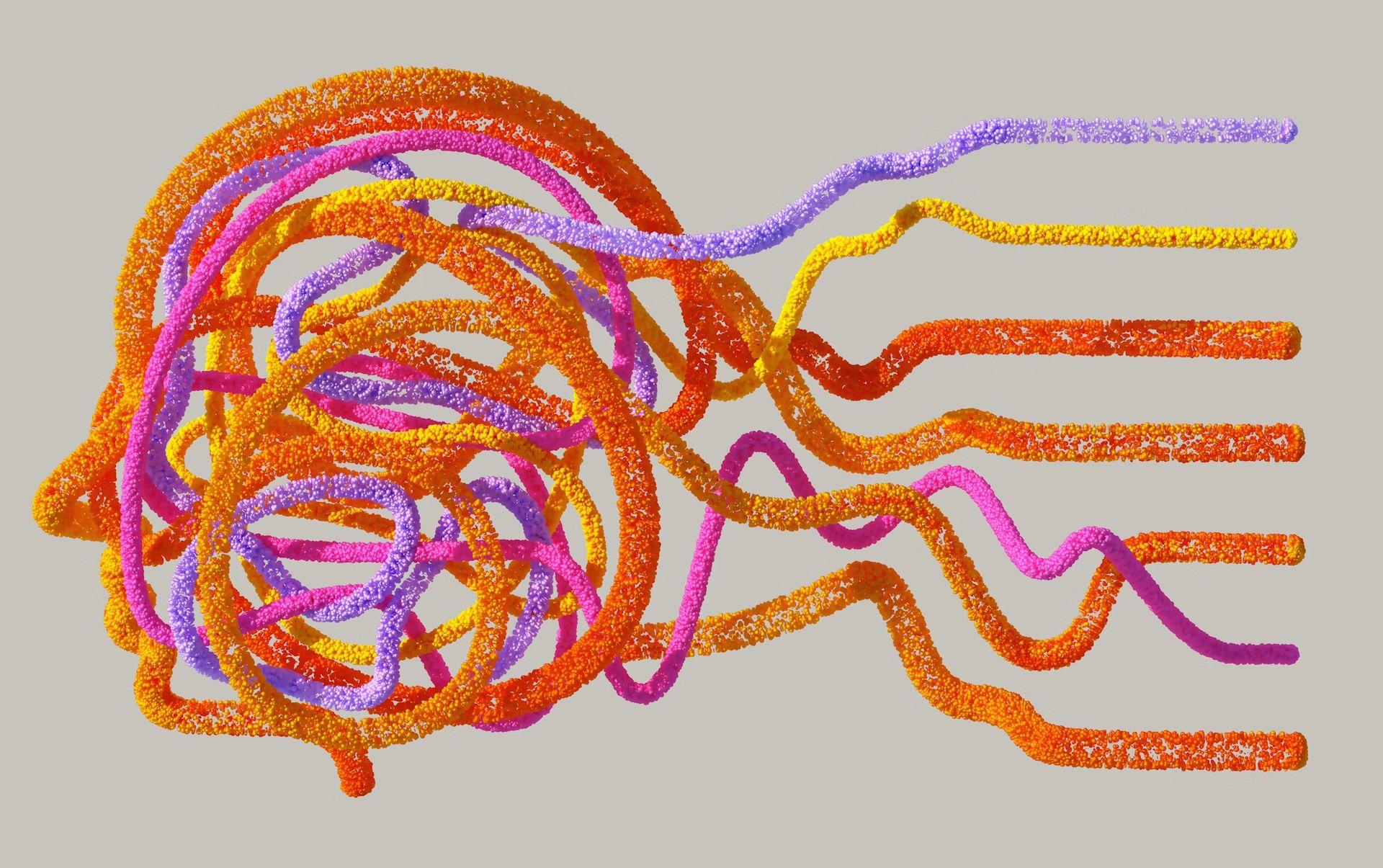
The procedure involves cross-referencing the information from the source against established truths. If the information proves to be even slightly incorrect, the source should be set aside in search of another. Huang underscores that AI’s role is not just to deliver answers but to conduct preliminary investigations to ascertain the most accurate responses.
For responses that bear significant importance, such as medical guidance, the head of Nvidia suggests that verifying information across various trusted sources is crucial. This implies that the AI responsible for generating answers must be capable of acknowledging when it lacks sufficient information to provide a response, when there is no clear consensus on the correct answer, or when certain information, like the outcome of future events, is not yet available.
Featured image credit: NVIDIA