- Researchers built AI that can learn tasks from text instructions and then communicate that knowledge to other AI systems. This eliminates the need for individual training for each AI, streamlining development.
- The AI network understands complete sentences, mimicking human interaction. This advancement in Natural Language Processing (NLP) allows AI to collaborate more effectively with humans.
- While not sentient, this AI communication opens the way for further exploration. It sparks discussions on AI’s potential benefits and drawbacks in society, including concerns about surpassing human control.
A team of researchers has developed an AI capable of communicating amongst themselves. This innovative model is designed to acquire knowledge and execute assignments from text-based directives, subsequently transferring this knowledge to other AI systems, according to the researchers. The details of this breakthrough and the AI’s evolution have been published in the journal Nature.
How did researchers accomplish that?
To evaluate its efficiency, the researchers enabled one AI to master a task using the instructions provided. This AI was then able to share its knowledge with another AI, termed a “sister” AI, enabling the latter to complete the given tasks without the need for previous training or experience. This advancement marks a significant leap forward for AI networks, demonstrating the immense possibilities offered by integrating natural language processing (NLP) into AI technology.
Researchers have advanced artificial intelligence by exploring agents capable of direct communication and mutual task learning. The breakthrough involves constructing an AI network trained solely on written instructions, which subsequently imparts its knowledge to a counterpart AI. This milestone hinges on harnessing natural language processing, enabling machines to comprehend and replicate human language naturally. The AI network comprises neural networks, intricate assemblies of machine learning algorithms modeled after the human brain’s neuronal structure.
The fundamental goal of natural language processing is to mimic human language in computers, enabling these machines to interpret and emulate text or spoken words with greater ease and naturalness. This concept is increasingly pertinent given the surge in AI developments, though achieving full viability remains a distant goal. The advent of AI capable of mutual communication represents a significant stride in this journey.
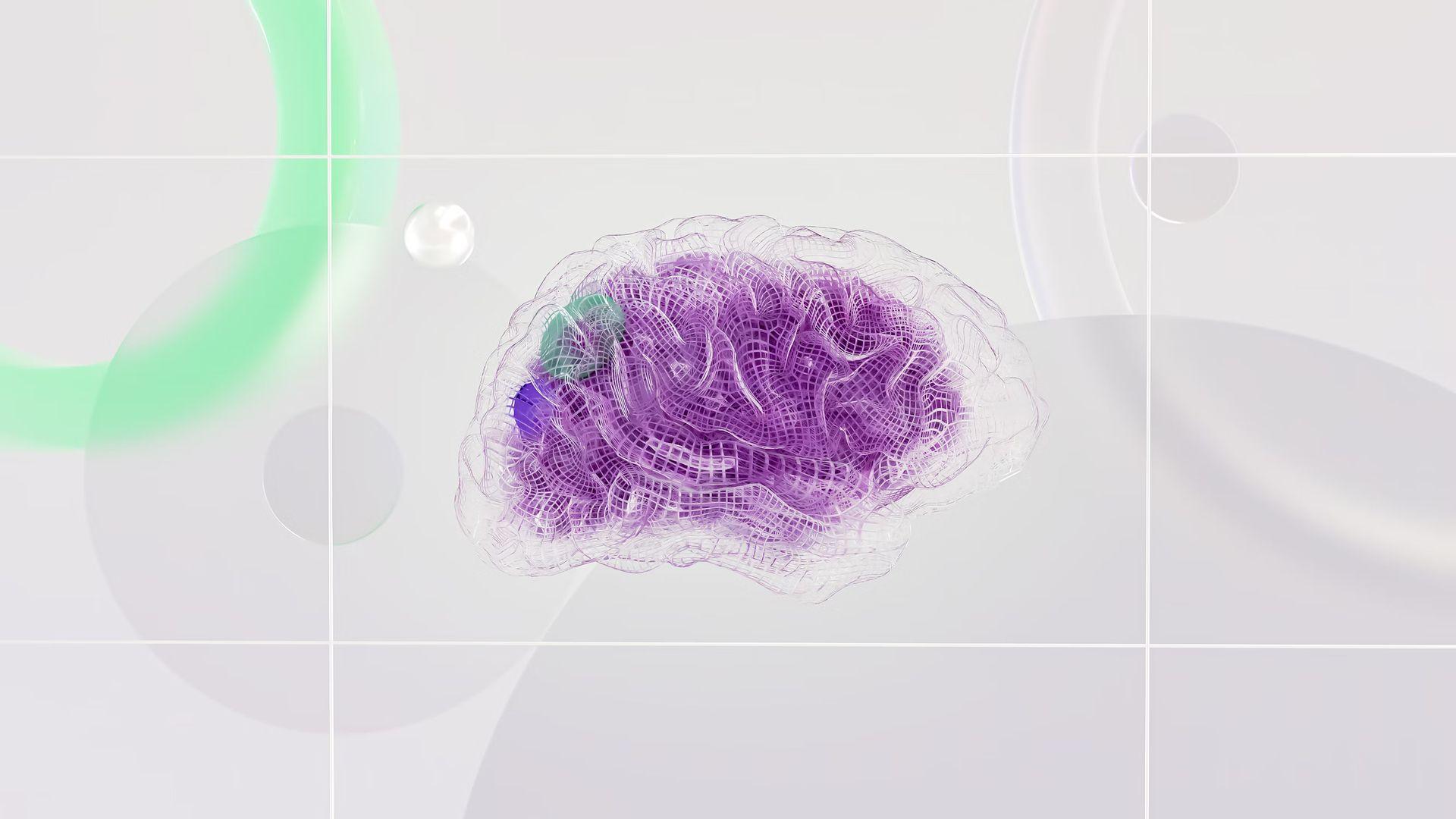
The composite AI, a “sensorimotor-recurrent neural network (RNN),” undergoes training on a repertoire of psychophysical tasks, responding to stimuli like light based on instructions conveyed through NLP. Despite lacking prior exposure or task-specific training data, the RNN achieves an impressive 83% accuracy in executing tasks guided by natural language instructions. Its comprehension of written sentences facilitates task execution, culminating in the articulation of outcomes via linguistic instructions to a sibling AI, enabling task execution without prior experience.
This breakthrough simplifies the process of developing AI models by eliminating much of the preliminary groundwork. Conventionally, each model requires individual training for specific tasks. However, a network wherein AI entities can share knowledge circumvents the necessity for such extensive initial training.
The scientists behind this network have achieved a milestone by enabling the model to process and react to complete sentences in a manner that mimics natural human interaction more closely. This represents a monumental leap in the capabilities of AI models, enhancing their potential to collaborate more seamlessly with human operators.
While AI systems communicating amongst themselves do not equate to creating an AI with human-like reasoning abilities, this development paves the way for new explorations in AI technology. It raises both excitement and apprehension, fueling debates about AI’s potential impacts on society, including speculative concerns about AI surpassing human control.
Featured image credit: Google DeepMind/Unsplash